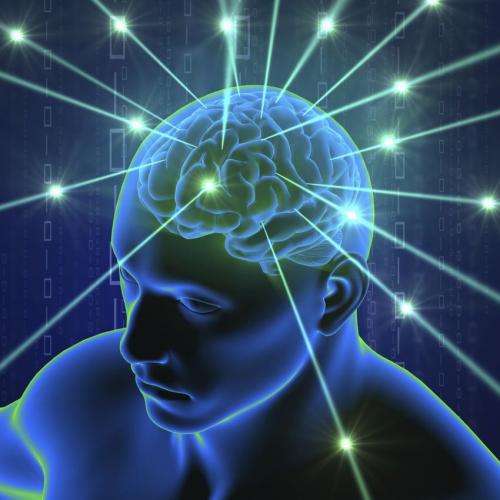
剑桥大学的科学家们已经证明,对人工智能系统施加物理约束——就像人类大脑必须在物理和生物约束下发展和运行一样——可以使其发展复杂生物大脑的特征,以解决任务
当大脑等神经系统组织自己并建立联系时,它们必须平衡相互竞争的需求。例如,需要能源和资源来在物理空间中发展和维持网络,同时优化网络以进行信息处理。这种权衡塑造了物种内部和跨物种的所有大脑,这可能有助于解释为什么许多大脑会趋同于类似的组织解决方案
该系统使用的不是真正的神经元,而是计算节点。神经元和节点的功能相似,每个节点都接受一个输入,对其进行转换,并产生一个输出,单个节点或神经元可能连接到多个其他节点,所有这些都是要计算的输入信息
然而,在他们的系统中,研究人员对系统施加了“物理”约束。每个节点在虚拟空间中都有一个特定的位置,两个节点越远,它们的通信就越困难。这类似于人类大脑中神经元的组织方式
研究人员给了该系统一个简单的任务来完成——在这种情况下,这是一个迷宫导航任务的简化版本,通常是在研究大脑时给老鼠和猕猴等动物的,它必须结合多条信息来决定到达终点的最短路线
团队选择这项特定任务的原因之一是,为了完成这项任务,系统需要维护许多元素——开始位置、结束位置和中间步骤——一旦学会了可靠地完成任务,就可以在试验的不同时刻观察到哪些节点是重要的。例如,一个特定的节点集群可以对完成位置进行编码,而其他节点则对可用路线进行编码,并且可以跟踪哪些节点在任务的不同阶段是活动的
最初,系统不知道如何完成任务并出错。但当它得到反馈时,它会逐渐学会更好地完成任务。它通过改变节点之间的连接强度来学习,类似于我们学习时脑细胞之间连接强度的变化。然后,系统一遍又一遍地重复该任务,直到最终学会正确执行
然而,对于他们的系统,物理约束意味着两个节点越远,就越难在两个节点之间建立响应反馈的连接。在人类大脑中,跨越巨大物理距离的连接形成和维护成本高昂
当系统被要求在这些约束条件下执行任务时,它使用了一些与真实人类大脑相同的技巧来解决任务。例如,为了绕过这些限制,人工系统开始开发集线器——高度连接的节点,充当在网络中传递信息的管道
然而,更令人惊讶的是,单个节点本身的响应曲线开始发生变化:换句话说,节点开发了一种“灵活的编码方案”,而不是每个节点都为迷宫任务的一个特定属性编码,比如目标位置或下一个选择这意味着,在时间的不同时刻,节点可能会因迷宫的各种特性而开火。例如,同一节点可能能够对迷宫的多个位置进行编码,而不需要专门的节点来对特定位置进行编码。这是复杂生物大脑中的另一个特征
合著者、剑桥大学精神病学系的Duncan Astle教授说,“这种简单的约束-很难将与人工系统相距甚远的节点连接起来,以产生一些相当复杂的特征。有趣的是,它们是像人类大脑这样的生物系统所共有的特征。我认为这告诉我们一些关于为什么我们的大脑是这样组织的基本信息。”。“了解人类大脑
该团队希望他们的人工智能系统能够开始阐明这些限制因素是如何形成人们大脑之间的差异的,并导致那些经历认知或心理障碍的人的差异
More surprising, however, was that the response profiles of individual nodes themselves began to change: in other words, rather than having a system where each node codes for one particular property of the maze task, like the goal location or the next choice, nodes developed a 'flexible coding scheme.' This means that at different moments in time nodes might be firing for a mix of the properties of the maze. For instance, the same node might be able to encode multiple locations of a maze, rather than needing specialized nodes for encoding specific locations. This is another feature seen in the brains of complex organisms.
Co-author Professor Duncan Astle, from Cambridge's Department of Psychiatry, said, "This simple constraint—it's harder to wire nodes that are far apart—forces artificial systems to produce some quite complicated characteristics. Interestingly, they are characteristics shared by biological systems like the human brain. I think that tells us something fundamental about why our brains are organized the way they are."
Understanding the human brain
The team is hopeful that their AI system could begin to shed light on how these constraints, shape differences between people's brains, and contribute to differences seen in those that experience cognitive or mental health difficulties.
Co-author Professor John Duncan from the MRC CBSU said, "These artificial brains give us a way to understand the rich and bewildering data we see when the activity of real neurons is recorded in real brains."
Achterberg added, "Artificial 'brains' allow us to ask questions that it would be impossible to look at in an actual biological system. We can train the system to perform tasks and then play around experimentally with the constraints we impose, to see if it begins to look more like the brains of particular individuals."
Implications for designing future AI systems
The findings are likely to be of interest to the AI community, too, where they could allow for the development of more efficient systems, particularly in situations where there are likely to be physical constraints.
Dr. Akarca said, "AI researchers are constantly trying to work out how to make complex, neural systems that can encode and perform in a flexible way that is efficient. To achieve this, we think that neurobiology will give us a lot of inspiration. For example, the overall wiring cost of the system we've created is much lower than you would find in a typical AI system."
Many modern AI solutions involve using architectures that only superficially resemble a brain. The researchers say their work shows that the type of problem the AI is solving will influence which architecture is the most powerful to use.
Achterberg said, "If you want to build an artificially-intelligent system that solves similar problems to humans, then ultimately the system will end up looking much closer to an actual brain than systems running on large compute clusters that specialize in very different tasks to those carried out by humans. The architecture and structure we see in our artificial 'brain' is there because it is beneficial for handling the specific brain-like challenges it faces."
This means that robots that have to process a large amount of constantly changing information with finite energetic resources could benefit from having brain structures not dissimilar to ours.
Achterberg added, "Brains of robots that are deployed in the real physical world are probably going to look more like our brains because they might face the same challenges as us."
"They need to constantly process new information coming in through their sensors while controlling their bodies to move through space towards a goal. Many systems will need to run all their computations with a limited supply of electric energy and so, to balance these energetic constraints with the amount of information it needs to process, it will probably need a brain structure similar to ours."